Table Of Content
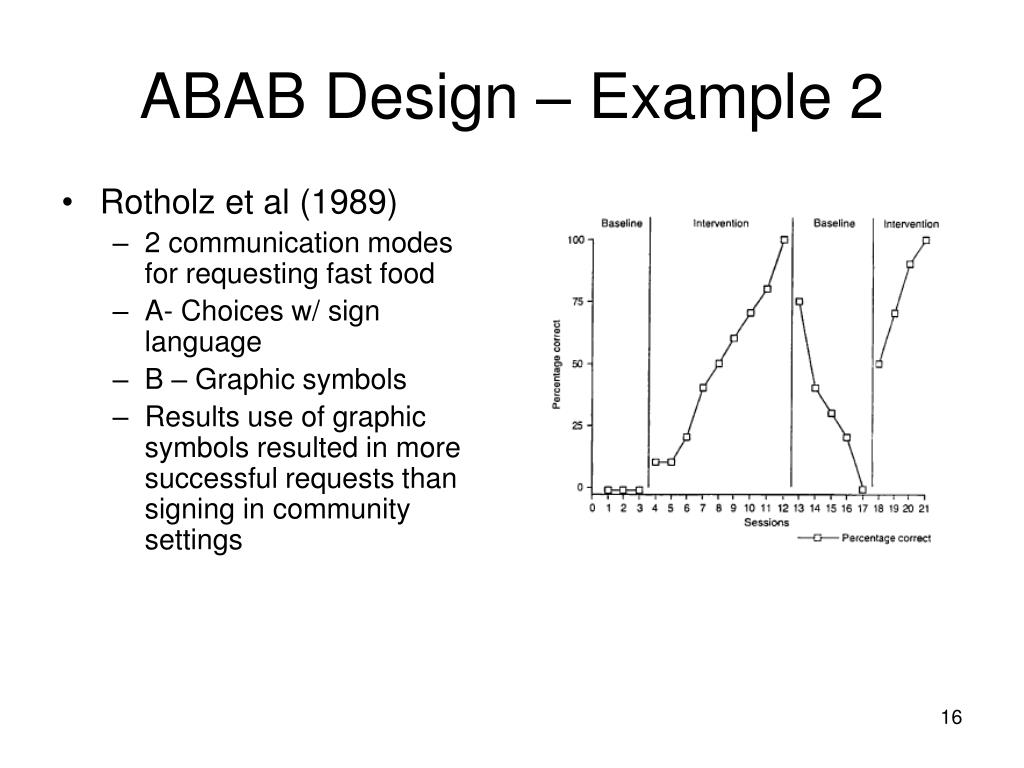
A traditional withdrawal design allows for comparisons over time between two baseline and two intervention conditions, though variations with additional A or B conditions can be utilized (e.g., ABABAB). For example, if the behavior of calling out in class follows the pattern in figure 1 in which baseline responding in A1 and A2 is significantly higher than responding during intervention phases B1 and B2, then we have strong evidence that a functional relationship exists. When the intervention is first put into place (B1) it shows a change in the frequency of the behavior. However, there is the possibility that other changes in the environment occurring at the same time are what caused the decrease in frequency. The withdrawal of the IV (A2) and resulting increase in responding makes the case that the other variables which may or may not still be in place become less likely to be a cause of the change in rate. The second implementation of the IV (B2) and resulting decrease of responding provides further evidence that changes in the DV are primarily due to the implementation of the IV.
Exploring the Science: Can Antibiotics Impact Autism Risk?
Consequently, variables unrelated to treatment may inadvertently cause behavior changes, which may be erroneously explained as being the result of treatment. In our analyses, we assumed that changes produced by confounding variables during the initial phase change would not be replicated, but we did not consider confounds that may operate within phases or across subsequent phase changes. As such, the issue of confounding variables remains unresolved and should be carefully considered in future research. To be included in our analysis, a graph had to include an ABAB design where A represented a baseline condition and B a treatment condition, be based on empirical data, and have a minimum of three data points per phase.
Understanding the Unique PDA Profile in Autism: A Comprehensive Analysis
ABAB-design studies are a good way to complete an initial investigation before a large-scale trial is completed. In an ABAB-design study, the intervention is reintroduced after a return to the baseline to see how strong the intervention was. ABAB and ABA are types of research protocols called single-subject experimental design (SSED).
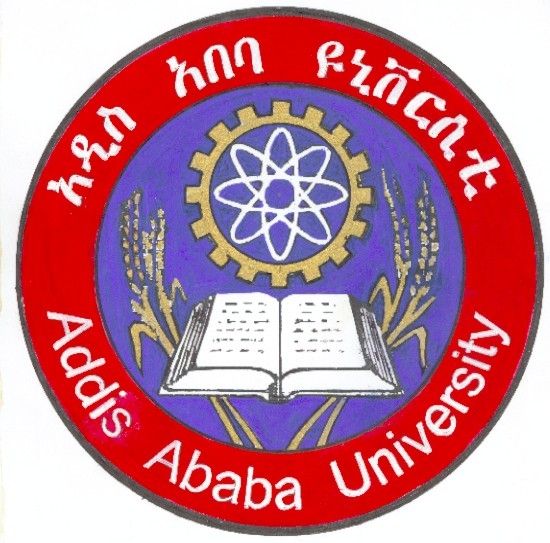
Single-Subject Research Designs
Hereafter, we will use the numbering in Table 1 to refer to each of the 15 data patterns individually. In the previous section, we showed the validity of the randomized AB phase design and the RT with respect to the Type I error for data containing unexpected linear trends. Another criterion for the usability of the RT for specific types of data sets, apart from controlled Type I error rates, is adequate power. In this section we focus on the power of the RT in the randomized AB phase design when the data contain unexpected linear trends. Previous research has not yet examined the effect of unexpected linear data trends on the power of the RT in randomized AB phase designs.
ABA vs. ABAB Design in Applied Behavior Analysis
More specifically, Tate et al. consider the AB phase design as a quasi-experimental design, and Vohra et al. even regard the AB phase design as a nonexperimental design, putting it under the same label as case studies. In contrast, the SCED classification by Logan, Hickman, Harris, and Heriza (2008) does include the AB phase design as a valid design. The SCD standards prioritizes visual analysis of the time-series data to assess the reliability and magnitude of intervention effects [29, 31, 40].
By carefully considering these factors, you can select the design that aligns with your research objectives and provides valuable insights into the effectiveness of interventions or the relationship between variables. Remember, the ultimate goal is to contribute to the advancement of knowledge and improve outcomes in the field of applied behavior analysis. Practitioners should also bear in mind that the AB design does not allow the demonstration of functional control. That is, the introduction of the independent variable may coincide with other life events that produce behavior changes.
Of course, this result is to be expected, because the size of the treatment effect ranged from 0 to 4 (in absolute value), which is a very large difference. The large amount of variance explained by the treatment effect size also accounts for the large standard deviations for the power levels of the other main effects (displayed in Tables 4–8 in the Appendix). To visualize the effect of the simulation factors on the RT’s power, we plotted the effect of each simulation factor in interaction with the size of the treatment effect (β2) while averaging the power across all other simulation factors in the simulation study in Fig.
One of the tools used to help answer the question of “what works” that forms the basis for the evidence in evidence-based practice is meta-analysis—the quantitative synthesis of studies from which standardized and weighted effect sizes can be derived. Meta-analysis methodology provides an objective estimate of the magnitude of an intervention's effect. One of the main problems of SSEDs is that the evidence generated is not always included in meta-analyses. Alternatively, if studies based on SSEDs are used in meta-analysis, there is no agreement on the correct metric to estimate and quantify the effect size. The AATD eliminates some of the concerns regarding multiple-treatment interference because different behaviors are exposed to different conditions. As in the multiple-baseline/multiple-probe designs, the possibility of generalization across behaviors must be considered, and steps should be taken to ensure the independence of the behaviors selected.
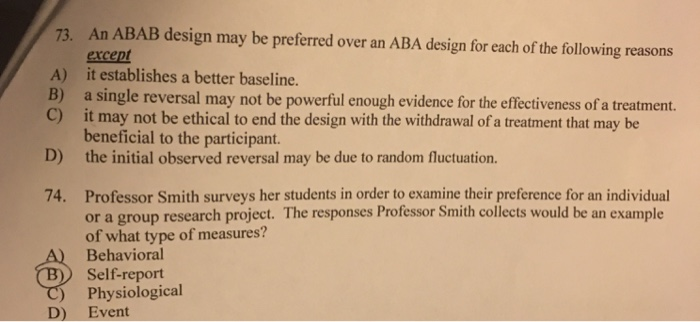
Choosing the Right Design for Your Study
Therefore, continuing the baseline condition until there is no visible trend helps build our confidence about the causal role of the treatment when it is introduced. The immediate decrease in the level of smoking for participant 1 when the treatment is introduced also implicates the treatment. We can also detect, however, an increasing trend in the early portion of the treatment condition.
Many researchers have argued that our research tools have become limited, particularly in the domain of behavioral health interventions [1–9]. The reasons for their arguments vary, but include an overreliance on randomized controlled trials, the slow pace and high cost of such trials, and the lack of attention to individual differences. In addition, advances in mobile and sensor-based data collection now permit real-time, continuous observation of behavior and symptoms over extended durations [3, 10, 11].
We showed that randomized AB phase designs can be analyzed with randomization tests to assess the statistical significance of the mean level changes and immediate changes in the outcome variable by using appropriate test statistics for each type of effect. The results of a simulation study showed that the power with which mean level changes and immediate changes can be evaluated depends on the specific type of data pattern that is analyzed. In any case, randomized AB phase designs should contain more than 30 measurement occasions to provide adequate power in the RT. Researchers should be aware that the randomized AB phase design generally has low power, even for large sample sizes. For this reason, we recommend that researchers use single-case phase designs with more power (such as randomized multiple-baseline designs or a serially replicated randomized AB phase design) whenever possible, as they have a higher statistical-conclusion validity.
Johnson County Middle School Robotics Team wins first place VEX Robotics Create Award in world competition - The Tomahawk
Johnson County Middle School Robotics Team wins first place VEX Robotics Create Award in world competition.
Posted: Wed, 26 Apr 2017 07:00:00 GMT [source]
Its applications span across diverse populations, including individuals with autism spectrum disorder, developmental disabilities, behavioral disorders, and other behavioral challenges. In this section we will discuss the power differences between the different types of data patterns in the simulation study. 3 show the main effects of the number of measurement occasions, the level of autocorrelation, the size of the A phase trend, the size of the B phase trend, and the effect size measure used, respectively, on the power of the RT. We will summarize the results concerning the main effects for each of these experimental factors in turn. Table 2 shows that by far the largest amount of variance was explained by the size of the treatment effect (β2).
No comments:
Post a Comment